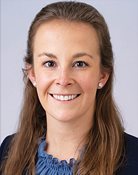
Fall 2021 - Vol. 16, No. 3
Social Determinants of Glycemic Control
A Retrospective Study
Mara Wilson, PharmD, BCACP
Ambulatory Pharmacist Clinician
Penn Medicine Lancaster General Health
Editor’s Note: A synopsis of this article appears in the print journal of September 2021, which was the final issue before the retirement of the Journal’s current Editor-in-Chief and Managing Editor.
Because of special plans for that issue, which republished several past articles that have particular relevance to the times in which we are living, there was insufficient space in the print edition of the Journal to publish the full article with the statistical analyses underlying its conclusions.
Here on our website we are able to publish the full article.
ABSTRACT
In order to identify social determinants of health that impact glycemic control in Type 2 diabetes, we conducted a retrospective, cross sectional analysis that compared three groups of patients based on their most recent hemoglobin A1C within the past year.
The three groups were defined on the basis of a hemoglobin A1C of ≤8%, >8% to 10%, or >10%. To be included, patients had to be at least 18 years of age, active in the diabetes registry, and managed by a Penn Medicine Lancaster General Health physician. Our objective was to identify those characteristics associated with the hemoglobin A1C of each predefined patient group.
We found that the odds of worsening glycemic control (a higher Hb A1C) were increased by male gender, African American race, Hispanic ethnicity, low financial class, barriers to medications, higher score on the patient health questionnaire-9 (PHQ9) or social vulnerability index (SVI), and higher no-show rate. In contrast, the odds of worsening glycemic control were decreased by greater age and level of education, including a high school or GED (general educational development) diploma, as well as vocational school, college, or higher education.
These results demonstrate that social determinants of health impact glycemic control. It is not enough to focus on glycemic control as a numerical target; we must identify which characteristics impact each individual patient, so we can connect them with the proper psychosocial and/or socioeconomic resources, and ensure they have the appropriate tools to manage their diabetes.
INTRODUCTION
The American Diabetes Association (ADA) has dedicated a specific section in the 2020 Standards of Medical Care in Diabetes to population health.
1 Although the number of patients with diabetes who achieve hemoglobin A1C goals has increased over the years, a substantial portion of the population fails to meet recommended targets.
1 The Centers for Disease Control and Prevention (CDC) describes social determinants of health (SDOH) as “conditions in the places where people live, learn, work, and play that affect a wide range of health risks and outcomes.”
2
There is ample literature that demonstrates the importance of SDOH specifically regarding diabetes management. These factors include financial barriers, food insecurity, lack of adequate housing, and medical comorbidities, most notably depression.
3,4,5
Financial distress impacts approximately half of the adult patient population with diabetes, with one in five patients reporting food insecurity,
6 which can lead to inadequate or sporadic consumption of inexpensive carbohydrates and processed foods, further complicating glycemic control.
7 Housing, especially homelessness, is associated with additional barriers beside food insecurities, including cost and storage of medications, and behavioral health problems.
1 In the latter regard, both untreated depression and failure to keep appointments are independent causes of a hemoglobin A1C >9%.
8
The challenges posed by financial limitations, depression, and the cost of medications, not only impact adherence to therapeutic regimens and impair glycemic control, but may go unrecognized.
4 In one study, two-thirds of patients who did not adhere to any of their medications due to cost had never discussed this problem with their provider.
9 Other studies found ethnic disparities in glycemic control, and an association with neighborhood support in the inner city.
10,11 Yet another study concluded that patients with type 2 diabetes in an urban primary care population with a higher level of neighborhood support had better glycemic control (A1C <7%).
12 Psychosocial concerns provide another barrier that has been identified in studies as a target to address in order to improve outcomes.
1
Our study sought to determine if patients with differing degrees of glycemic control – as determined by hemoglobin A1C – are stratified by different social determinants of health.
METHODS
Study design and population
This study was a retrospective analysis that compared three groups based on their most recent hemoglobin A1C within the past year. The three predefined groups consisted of patients with a hemoglobin A1C of ≤8%, >8% to 10%, and >10%. Patients included were 18 years of age or older, had a diagnosis of diabetes mellitus, were active in the electronic health record’s (EHR) diabetes registry, and were managed by a Penn Medicine Lancaster General Health Physicians (LGHP) Primary or Specialty Care practice provider.
Elements of the risk assessment measures (RAM) score included a series of questions regarding barriers to care, cognitive functional status, health literacy, depression based on patient health questionnaire-9 (PHQ9) score, exercise, self-efficacy, and social connection.
The CDC’s Social Vulnerability Index (SVI) uses U.S. Census data to determine the social vulnerability of every census tract (subdivisions of counties for which the Census collects statistical data). The SVI ranks each tract on 15 social factors, and groups them into four related themes: socioeconomic status, household composition and disability, minority status and language, and housing type and transportation.
13 Each tract receives a separate ranking for each of the four themes, as well as an overall SVI ranking to provide a comprehensive score. Values are represented as percentiles, with an increase in percentile indicating a more vulnerable census tract. Each tract was compared to data for the state of Pennsylvania.
Data collection
Patient demographics and laboratory values were extracted from the EHR and the diabetes registry. Patients were deemed active in the registry if a diagnosis code of diabetes was on their problem list, with either an encounter diagnosis or invoice diagnosis in the previous two years. Diagnosis codes were based on definitions from the National Institute of Health Value Set Authority Center. Deceased patients and test patients were not included in the registry.
For a patient to be included in the study, their most recent hemoglobin A1C had to be within the year prior to the date of data extraction. This number was used to assign patients to each of the three groups, though data were retrospectively pulled from the previous three years. All data variables included were the most recent value at the time of extraction. Patients were included in the study even if all data variables were not complete.
Data analysis
The primary objective of this study was to identify characteristics associated with having a hemoglobin A1C within each predefined patient group. We hypothesized that in Lancaster County there are SDOH associated with the level of glycemic control in a diabetic population.
Accordingly, descriptive summaries were utilized to show univariate associations with the three patient groups based on the patients’ most recent hemoglobin A1C value. Generalized ordered logit models were performed to identify characteristics associated with having an A1C of ≤8% compared with >8–10%, or >10% compared with an A1C ≤8% or 8–10%, while controlling for covariates. Geographic information software (GIS) was used to identify geospatial associations and clustering by census tract in any of the regions within the health system to identify the location of populations that were potentially at an increased risk for inadequate glycemic control. All analyses were performed using Stata Version 16.1.
RESULTS
Baseline Characteristics
Baseline characteristics are shown in Table 1. Overall, patients with an A1C <8% tended to be older, white, non-Hispanic, and English speaking. There was also a higher portion of patients with a vocation, college, or higher level of education in the ≤8% group compared with the other groups. Patients with an A1C >10% were more likely to be younger, and there was a higher proportion who were African-American and Hispanic compared with the other groups. The group with A1C >10% also had a higher proportion of patients with Commercial or Medicaid insurance, as well as those who were uninsured, compared with the other groups.

Among patients with completed RAM (risk assessment measures) questionnaires, the group with A1C >10% had a higher portion who reported barriers to care, cognitive difficulty, limited health literacy, higher PHQ9 scores, and both poorer self-efficacy and social connection, compared with the other two groups (Table 2). Based on Census tract from the neighborhood where the patient resides, the >10% group was in the higher percentile for vulnerability regarding socioeconomic status, household composition & disability, minority status & language, housing type & transportation, as well as overall SVI, compared with those in the other two groups (Table 3).
Adjusted Analysis
Generalized ordered logit models were performed to identify characteristics associated with an A1C of >8–10% or >10% compared with ≤8%, as well as an A1C >10% compared with ≤8% or 8–10%, while controlling for covariates as shown in Tables 4-7. There were two models performed for the final analysis, the second model also including the no-show rate for office visits.
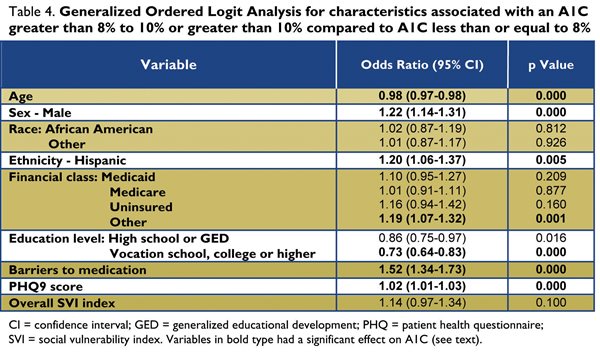
For the first model, characteristics shown to increase the odds of being in the >8% to 10% or >10% group compared with ≤8% were male gender, Hispanic ethnicity, “other” financial class defined as other government insurance programs, barriers to medications, and higher PHQ9 score
(Table 4). In contrast, increased age as well as high school diploma or general educational development (GED) and vocational school, college, or higher, decreased the odds of being in the >8% to 10% or >10% group compared with the ≤8% group (Table 4).
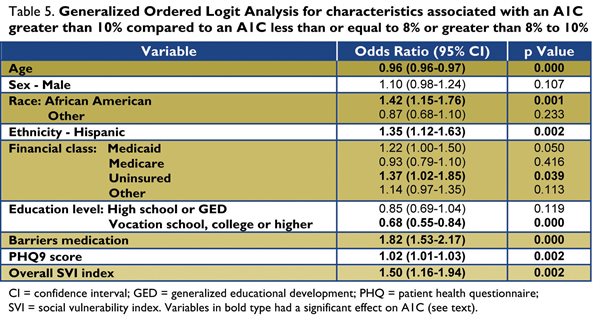
Characteristics that increased the odds of being in the >10% group compared with the ≤8% or >8% to 10% groups were African American race, Hispanic ethnicity, no insurance, barriers to medications, higher PHQ9 score, and higher SVI score (Table 5). In contrast increased age, and vocational school, college or higher education, decreased the odds of being in the >10% group compared with the ≤8% group or >8% to 10% group (Table 5).
For the second model, which included variables from the first model in addition to no-show rate, characteristics that were shown to increase the odds of being in the >8% to 10% or >10% group compared with the ≤8% group were male gender, barriers to medications, higher PHQ9 score, and increased no-show rate (Table 6). In contrast, increased age and vocation school, college, or higher decreased the odds of being in the >8% to 10% or >10% group compared to ≤8% (Table 6). Characteristics that were shown to increase the odds of being in the >10% group compared with the ≤8% or >8% to 10% groups were barriers to medications, higher PHQ9 scores, increased SVI score, and no-show rate. In contrast, increased age and vocational school, college or higher education decreased the odds of being in the >10% group compared with the ≤8% or >8% to 10% groups.
DISCUSSION
This study identified social determinants of health that impact glycemic control. Younger age was associated with worse glycemic control which is similar to previous findings in the literature.
14,15,16 There are several hypotheses as to why older adults have better glycemic control than younger diabetics. Older patients may have better adherence and are potentially more motivated to care for their diabetes while younger patients may not be as accepting, or may disregard the severity and potential for complications of diabetes, and are therefore less likely to engage in proper care.
14-17 Additionally, younger adults with diabetes tend to be more obese, resulting in an increase in insulin resistance and worse glycemic control, and a need for more intensive regimens compared with older patients.
Patients with higher levels of education were more likely to have better glycemic control, and better health outcomes in general, likely due to a higher instance of socioeconomic stability.
18 Pampel et al. also concluded those with a higher level of education are more likely to engage in preventive health measures such as proper diet and exercise, which also improve glycemic control.
19
RAM elements, specifically PHQ9 scores and barriers to medications, were shown to have a significant association with glycemic control. Our study found increasing PHQ9 scores were associated with worse glycemic control. Studies by others have shown that medical comorbidities, most notably depression, impact one’s ability to manage
diabetes.
20,4 This finding emphasizes the need to ensure patients are connected with mental health resources and support, along with focusing on their glycemic control. Unsurprisingly, barriers to care, specifically to obtaining medications, were also associated with worsening glycemic control.
An increase in social vulnerability, which is a composite of socioeconomic status, household composition & disability, minority status & language, and housing type & transportation, was associated with a higher A1C, illustrating that the neighborhood where a patient resides has an overall impact on glycemic control. These finding are similar to previously mentioned studies which have shown the impact of neighborhood support, ethnic disparities, financial barriers, food insecurity, and lack of adequate housing on glycemic control.
Race and ethnicity have been previously identified in the literature to significantly impact glycemic control. Such findings have been attributed to varying disparities such as differences in socioeconomic status and culture, but the exact relationship is still an area of research.
5,21,22 When including no-show rate, race and ethnicity were not found to be statistically significant. This finding infers that no-show rate seems to be a more pronounced factor for impacting glycemic control than race or ethnicity; it potentially also suggests that certain racial and ethnic groups have higher no-show rates than others. When running further analyses for race, ethnicity, and no-show rate, African Americans and Hispanics have much higher no-show rates than others. However, despite higher no-show rates in certain groups, an individual’s high no-show rate increases the odds of worse glycemic control regardless of race or ethnicity. Clinically, it is important to recognize the differences between groups to improve quality of care.
Our study had some limitations. The retrospective nature of this study limited data collection to what was available in the EHR. Also, responses to the RAM questionnaire may not have been up to date to reflect current A1C. Some of the variables included in our study were not completed by each patient, most commonly the RAM questionnaire.
Findings from this study support the need to connect patients with psychosocial services in conjunction with medical management. A multi-faceted approach is imperative in order to identify which resources would be most valuable for each patient. Access to resources for behavioral health and socioeconomic barriers play a significant role in a patient’s engagement in their own care, and should be addressed along with medical management to improve glycemic control. Our study found a high portion of patients had incomplete RAM questionnaires within the EHR. As a health system, this highlights an opportunity for improvement to increase utilization of this tool to ensure we are identifying social determinants that may impact the patient’s ability to access and engage in care.
CONCLUSION
As previously published by others, and further supported by the results of this study, SDOH impact glycemic control. The results of our study demonstrate that in managing patients with diabetes it is insufficient to focus only on glycemic control; it is vital to identify the social characteristics of individual patients, so they may be connected with the psychosocial and/or socioeconomic resources they need, thus ensuring they will have the appropriate tools to manage their comorbidities.
ACKNOWLEDGEMENTS
Natalia Tarasiuk, PharmD, BCACP – Ambulatory Pharmacist Clinician; Lauren Enser, PharmD, BCACP – Ambulatory Pharmacist Clinician; Chelsea Cunningham, PharmD, BCACP – Ambulatory Pharmacist Clinician; Michael Horst, PhD, MPHS, MS – Director, Research Biostat & Quality; Christopher T. Addis, MD, FACP, MBA – Chairman, Department of Medicine; Jeffrey R. Martin, MD, FAAFP – Chair, Family and Community Medicine; Mary Papadoplos, MHA, RD, CDCES, LDN – Director, Medicine Service Line; Matthew A. Sauder, MD – Managing Physician, Diabetes and Endocrinology Specialists; Deepika Pradhan Shrestha, MD – Division Chief, Diabetes and Endocrinology Specialists.
REFERENCES
1. American Diabetes Association. 1. Improving care and promoting health in populations: standards of medical care in diabetes 2020.
Diabetes Care 2020;42: S7-12.
2. Centers for Disease Control and Prevention. Social Determinants of Health: Know What Affects Health.
https://www.cdc.gov/socialdeterminants/index.htm (accessed 2019 July 26).
3. Hill JO, Galloway JM, Goley A, et al. Socioecological determinants of prediabetes and type 2 diabetes.
Diabetes Care 2013;36: 2430-2439.
4. Katon WJ, Lin EHB, Von Korff M, et al. Collaborative care for patients with depression and chronic illnesses.
N Engl J Med 2010;363: 2611-2620.
5. Walker RJ, Strom Williams J, Egede LE. Influence of race, ethnicity and social determinants of health on diabetes outcomes.
Am J Med Sci 2016;351: 366-373.
6. Patel MR, Piette JD, Resnicow K, et al. Social determinants of health, cost-related nonadherence, and cost-reducing behaviors among adults with diabetes: findings from the national health interview survey.
Med Care 2016;54: 796-803.
7. Seligman HK, Schillinger D. Hunger and socioeconomic disparities in chronic disease.
N Engl J Med 2010;363: 6-9.
8. Rivich J, Kosirog ER, Billups SJ, et al. Social and psychosocial determinants of health associated with uncontrolled diabetes in a federally qualified health center population.
Diabetes Spectrum 2019;32: 145-151.
9. Piette JD, Heisler M, Wagner TH. Cost related medication underuse among chronically ill adults: the treatments people forgo, how often, and who is at risk.
Am J Public Health 2004;94: 1782-1787.
10. Del Carpio RO, Stewart SH. Association between social determinants of health and glycemic control in an inner city primary care population.
Social Medicine 2016;10: 93-98.
11. Quandt SA, Bell RA, Snively BM, et al. Ethnic disparities in glycemic control among rural older adults with type 2 diabetes.
Ethnicity & Disease 2005;15: 656-663.
12. Del Carpio RO, Stewart SH. Association between social determinants of health and glycemic control in an inner city primary care population.
Social Medicine 2016;10: 93-98.
13. Agency for Toxic Substances and Disease Registry. CDC’s Social Vulnerability Index (SVI).
https://svi.cdc.gov/index.html (accessed 2020 May 26).
14. Toh M, Wu C, Leong H. Association of younger age with poor glycemic and cholesterol control in Asians with Type 2 diabetes mellitus in Singapore.
J Endocrinol Metab 2011;1: 27-37.
15. El-Kebbi IM, Cook CB, Ziemer DC, et al. Association of younger age with poor glycemic control and obesity in urban African Americans with type 2 diabetes.
Arch Intern Med 2003;163: 69-75.
16. Comellas M, Marrero Y, George F, et al. Age and glycemic control among adults with type 2 diabetes in the United States: an assessment from the national health and nutrition examination survey (NHANES) 2013–2014.
Diabetes Metab Syndr 2019;13: 3069-3073.
17. Glasgow RE, Hampson SE, Strycker LA, et al. Personal-model beliefs and social-environmental barriers related to diabetes self-management.
Diabetes Care 1997;20: 556-561.
18. Montez JK, Zajacova A. Explaining the widening education gap in mortality among U.S. white women.
J Health Soc Behav 2013;54: 166-182.
19. Pampel FC, Krueger PM, Denney JT. Socioeconomic disparities in health behaviors.
Annual Rev Soc. 2010;36: 349-370.
20. Walker RJ, Strom Williams J, Egede LE. Influence of race, ethnicity and social determinants of health on diabetes outcomes.
Am J Med Sci 2016;351: 366-373.
21. Parrinello CM, Rastegar I, Godino JG, et al. Prevalence of and racial disparities in risk factor control in older adults with diabetes: the atherosclerosis risk in communities study.
Diabetes Care 2015;38: 1290-1298.
22. Puckrein GA, Egan BM, Howard G. Social and medical determinants of cardiometabolic health: the big picture.
Ethnicity & Disease 2015;25: 521-524.