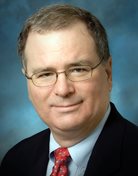
Winter 2018 - Vol. 13, No. 4
The End of Radiology?
Artificial Intelligence (AI) in Imaging
Leigh S. Shuman, M.D.
Radiologist
INTRODUCTION
At the annual meeting of the American College of Radiology in 2016, noted oncologist and health care policy expert Ezekiel Emanuel, M.D., caused a considerable stir with his keynote lecture titled “The End of Radiology?” In his talk, which was subsequently published in the
Journal of the American College of Radiology,
1 Emanuel outlined three threats to the future of the specialty:
1) The move away from hospital-based care to the outpatient setting, and an anticipated decrease in utilization of medical tests, especially imaging;
2) Ongoing efforts to reduce costs, which will inevitably drive reductions in reimbursement for imaging studies beyond the already significant reductions that have occurred over the past 10-15 years;
3) Machine learning, which he termed the “ultimate threat” to radiology. It will become a powerful tool in the next 10-15 years, and he believes it could “end radiology as a thriving specialty.”
1
Just to be sure the rest of the medical community got the message, Dr. Emanuel made essentially the same point in an article he coauthored in the
New England Journal of Medicine. 2 The non-medical world has picked up on this theme, and a recent article in the lay press had the provocative title, “If You Look at X-rays or Moles for a Living, Artificial Intelligence (AI) is Coming for You!”
3 All these comments have left many young radiologists questioning their choice of specialty, and has led to concern that medical students will no longer choose radiology as a specialty for fear that it will follow the job of buggy whip maker into oblivion. How realistic are these fears, and what is the promise and peril of the rapid development of AI for imaging over the past few years?
DEFINITIONS
The phrase
Artificial Intelligence (AI) was first coined in 1956 to describe the ability of machines to perform tasks that have typically needed human intelligence. With the rapid and exponential increase of computers’ processing power, and their decreasing cost, tools for
computer-aided diagnosis (CAD) became widely available in the late 1990s, particularly for the interpretation of mammograms. This software was trained to look for certain features in the images, such as calcifications or areas of increased density, and to flag them for further scrutiny by the interpreting radiologist. These tools had to be programmed with specific rules about what features to look for, and – once programmed – were no smarter reviewing their millionth mammogram than their first one.
Machine learning, on the other hand, means the computer can learn to do things it was not explicitly programmed to do at the outset. In this paradigm, the software is presented with a set of known examples of what is being sought, and develops its own set of rules about what to look for. The more examples it analyzes, the better it gets at recognizing the characteristics that distinguish normal from abnormal. Unlike conventional CAD software, the process is dynamic, and improvement over time is almost inevitable.
In the past few years, the tremendous growth in computational power found in high-end graphics cards and parallel processing, has allowed the creation of
deep learning tools. These techniques make use of convolutional neural networks (CNNs) that mimic some organizational features of the human brain, with multiple layers of processors similar to the vertical organization of neurons seen in parts of the neuraxis.
It is important not to overlook the word mimic in the discussion, as none of the tools developed so far comes close to the organizational complexity of the human brain. The tools can perform certain narrow tasks, like looking for nodules or areas of bleeding, but they are far from so-called
general AI, which would perform the full spectrum of human cognitive activities, not just a few key maneuvers. A full discussion of the differences between AI, machine learning, and deep learning, and some of the methodologies behind deep learning algorithms is available elsewhere.
4
IMAGE ANALYSIS
The bulk of the radiologist’s workday is spent viewing images, looking for deviations from normal anatomy, and interpreting the significance of those observations. Such work has become much more challenging with the explosion in the number of images most radiologists see in a day. In the era before cross-sectional imaging modalities such as CT, MRI, and ultrasound were widely available (the 1970s, when this author began in the field), radiologists might have viewed about 50 studies each day, with most consisting of two or three images each. A fluoroscopic study might have had 20 or 30 images, and a complex angiogram a few dozen, or even 100 images.
Today, the same physician may read far greater numbers of CT and MR exams. The average CT study has 200-500 images, and MRI exams typically have even more. Human ability to look for small abnormalities in this vast amount of visual information is often overwhelmed, and most of the images are normal, making finding the pathology akin to finding the proverbial needle in a haystack. Demands for ever greater productivity, longer working hours, and the demand for 24/7/365 services has increased observer fatigue, further worsening performance. And it is precisely here that computers excel: searching large data sets very rapidly, and identifying subtle variations.
Early approaches to AI have been used in radiology for several decades, especially in CAD programs that aid interpretation of mammographic screening exams. Although the number of images in each study is small (typically four), the radiologist may be viewing several hundred exams each day.
The findings that suggest cancer are very subtle, and are often only apparent when multiple studies are compared over time. The early CAD tools have generally been disappointing to most experienced mammographers,
5 because although they highlight areas of calcification or increased density for further analysis by the radiologist, they typically cannot compare one study with another and look for change. They tend to overcall, generally a desirable trait for screening exams, but they rarely detect important findings that the radiologist hasn’t noticed. Most importantly, they are not capable of learning, and do not become more accurate over time. Disappointment with these early systems has contributed to the skepticism that some radiologists feel about the new generation of AI machine-learning tools.
Several such tools capable of helping interpret images have now been reported, though very few have reached general commercial availability as of this writing. Examples include systems that identify critical findings such as pneumothorax,
6 lung nodules,
7 and large-vessel occlusions in the brain.
8 In all these instances, the software is complementary for the radiologist. Studies are prescreened by the software for significant findings, then moved up the interpretation work list to bring them to the radiologist’s attention more quickly. The key findings are annotated to aid the radiologist in more rapidly interpreting the entire exam, and notifying those directly caring for the patient.
The accuracy of these programs in identifying specific findings equals or sometimes exceeds that of experienced radiologists. Other software has been used not only to identify specific organs such as the liver or prostate on imaging studies, but also to identify masses within them, and then to characterize the likelihood the masses contain malignancy.
9 Still other programs focus on specific disease states, such as tuberculosis, and attempt to identify chest radiographs with a high probability of this disease.
10
Mammographic imaging, where the use of computers to aid interpretation began, now presents a host of new challenges with the advent of digital breast tomosynthesis (DBT), which has proven superior to conventional digital mammography for diagnosing early breast cancer, while reducing false positive mammograms with no increase in radiation dose. However, DBT has substantially increased the number of screening images that the radiologist must view for each patient, making new AI tools even more important.
11
In sum, the techniques outlined above are exciting developments, but are a long way from reading an entire imaging study and providing a report.
NON-INTERPRETIVE TASKS
The job of the radiologist does not begin or end with interpretation of images, and AI tools also have great potential to improve and speed up important tasks before and after images are obtained and interpreted.
12 A complex imaging study such as an abdominal MRI scan must have a protocol created in advance to determine the individually appropriate imaging sequences based on the specific information being sought about the patient’s suspected pathological condition, and any prior imaging studies for comparison. Deep learning tools have been developed to automate and streamline this process.
13 At the stage of image acquisition, software that uses AI has been developed to speed and optimize the reconstruction of cross-sectional images to provide more accurate diagnoses.
14 This can not only shorten examination times, but also lower the radiation dose needed to obtain diagnostic-quality images.
After the images are obtained, radiologists often struggle to get accurate and meaningful clinical information to aid in the interpretation of the study. Most referring providers lack the time or inclination to give the radiologist much or any useful information, which makes it extremely helpful to have EMR access integrated into the radiologist’s workstation. Several vendors now find and present the most useful information in the EMR to the reading radiologist, by using natural language processing (NLP) to parse free-text information, such as progress notes and consults.
15 For example, when reading an MRI of the brain, the most recent neurology consult would be extracted from the EMR and presented first to the radiologist, along with the most recent emergency department visit, notes that mention conditions such as headache, and the highlighted results of the most recent neuroimaging studies performed on that patient. Application of deep learning algorithms to NLP promises to make this process even more robust.
16 Obviously, radiologists are not the only ones who would benefit from tools that allow rapid extraction of key information from the bloated EMR data.
CHALLENGES
While AI has great promise in imaging, a number of challenges must be overcome as the field moves forward. Although the hype suggests that AI is mature, and the demise of radiologists is just around the corner, the reality is a bit more sobering.
One of the biggest problems is the need for curated data on which to train the machine- learning algorithms. There must be a set of cases where key findings are labeled, and the truth of the interpretation known. This at first sounds straightforward, but in the vast majority of cases, the gold standard of interpretation falls far short of pathologic proof. In breast imaging, for example, it may be easy to find a group of cases with biopsy-proven breast cancer, and put them through the computer, but not all cases that receive a benign diagnosis have microscopic confirmation, and they require many years of follow-up to be reliable. Similarly, a set of cases with pulmonary emboli on CT relies on human interpretation of the studies, as virtually all of the cases in the training set lack proof that emboli are in fact present or absent. Clear-cut examples pose no problems, but in studies with ambiguous interpretations and no pathological confirmation, the best standard one can hope for is expert consensus, which may be wrong. The history of imaging techniques that performed well in detecting disease after learning from a set of known positive examples, but then failed miserably at screening, is long and not pretty. Thermography for detecting breast cancer is a classic and instructive example from long ago.
As AI techniques become more and more complex, there comes a point where human beings can no longer understand just how the AI algorithm is reaching its conclusions. Can and will we then trust those conclusions?
17 An illustrative example from the military demonstrates the problem. The Pentagon developed an AI tool to detect camouflaged tanks in groups of trees. The computer was trained using a set of 50 photographs with tanks and 50 pictures with no tanks in a forest and proved to be 100% accurate in identifying the tanks on the remaining 100 test photos once it had learned how to spot the tanks.
Alas, it failed completely in the field. As the computer was incapable of explaining what it was doing, it was only after extensive analysis that it was discovered that the training photos with tanks in the woods were taken on a sunny day, while those with no tanks were obtained on cloudy days. The computer hadn’t learned to identify tanks in the trees at all, just to separate sunny from cloudy days.
18 In addition, because the deep-learning algorithms do not function in the same way as the human brain, they make very different kinds of errors than humans. Self-driving cars don’t fall asleep at the wheel or get distracted by texting, but they may confuse a billboard with an impending crash and apply emergency braking, a mistake even a new driver wouldn’t make.
19 Errors made in analyzing imaging studies may be similarly unexpected and unanticipated.
There are also numerous legal and regulatory hurdles to overcome. How will studies read by machines be paid for? If the machines make a mistake, who is liable, the manufacturer, the supervising radiologist, the institution that has deployed the software, or some combination of all of the above? These issues will need to be resolved if AI tools are going to achieve widespread clinical use.
CONCLUSION
Although the lay press and some radiology websites are filled with gushing testimonials about the coming of AI and the death of the specialty, the reality is more complicated. There are far more articles about the possible future of AI than there are actual successful applications of AI. We are still a very long way from computer systems that perform all the functions of the radiologist and do so in a reliable and consistent fashion. Even the tech industry has had some recent stumbles in their rush to apply this technology.
20
Nonetheless, radiologists would be naïve to hide their heads in the sand and pretend the day is never coming when their role will change radically. The American College of Radiology has formed a Data Science Institute, the goal of which is to help foster research on productive applications of AI and encourage the radiology community to guide and support the process, not avoid it. The American Medical Association has recently released a statement about AI in medicine.
21 Interestingly, they have chosen to change what the initials AI stand for, by substituting the term
augmented intelligence for
artificial intelligence to indicate the (hopefully) complementary roles of computers and human intelligence.
Within the radiology literature, one can find articles that lean toward the doom and gloom scenario suggested by Dr. Emanuel
22,23 along with those that take a more balanced view and suggest that AI will change, but not eliminate, the human factor in imaging.
24,25 What is clear is that change is coming very rapidly; the radiology community must become comfortable in the AI world, and use these tools and their own skills to remain valuable members of the health care team.
REFERENCES
1. Chockley K, Emanuel EJ. The End of Radiology? Three Threats to the Future Practice of Radiology.
J Am Coll Radiol 2016;13:1415-1420
2. Obermeyer Z, Emanuel EJ. Predicting the Future — Big Data, Machine Learning, and Clinical Medicine
N Engl J Med 2016; 375:1216-1219
3. Molteni M. If you look at x-rays or moles for a living, AI is coming for your job.
Wired Jan. 25, 2017. Accessed July15, 2018
4. Chartrand G, Cheng PM, Vorontsov E, et al. Deep Learning: A Primer for Radiologists.
RadioGraphics 2017; 37:2113–2131
5. Kohli A, Jha S. Why CAD failed in mammography.
J Am Coll Radiol 2017;15:535-537.
6. Li X, Digumarthy S, Kalra M, Li Q, et al. Deep learning algorithm for rapid automatic detection of pneumothorax on chest CT. Presented at the American Roentgen Ray Society, April 27, 2018.
7. Lo SB, Freedman MT, Gillis LB, et al. Computer-aided detection of lung nodules on CT with a computerized pulmonary vessel suppressed function.
American Journal of Roentgenology. 2018;210: 480-488.
8. Barreira C, Bouslama M, Lim J, et al. E-108 Aladin study: automated large artery occlusion detection in stroke imaging study – a multicenter analysis.
Journal of NeuroInterventional Surgery 2018;10:A101-A1.
9. Yasaka K, Akai H, Abe O, et al. Deep learning with convolutional neural network for differentiation of liver masses at dynamic contrast- enhanced CT: A preliminary study.
Radiology 2018;286:887-896
10. Lakhani P, Sundaram B. Deep learning at chest radiography: Automated classification of pulmonary tuberculosis by using convolutional neural networks.
Radiology 2017;284:574-582.
11. Salama RK, Chan HP, Hadjiiski L, et al. Mass detection in digital breast tomosynthesis: Deep convolutional neural network with transfer learning from mammography. Med Phys 2016; 43(12): 6654–6666.
12. Lakhani P, Prater AB, Hutson RK, et al. Machine learning in radiology: Applications beyond image interpretation.
J Am Coll Radiol 2017;15:350-35
13. Sohn JH, Trivedi H, Mesterhazy J, et al. Development and validation of machine learning based natural language classifiers to automatically assign MRI abdomen/pelvis protocols from free-text clinical indications. Paper presented at: Society of Imaging Informatics in Medicine, Annual Meeting; June 2017; Pittsburgh, PA.
14. Chen H, Zhang Y, Zhang W, et al. Low-dose CT via convolutional neural network.
Biomed Opt Express 2017;8:679-94.
15. Imaging Fellow, Change Healthcare. IBM Watson Imaging Patient Synopsis 1.0, IBM Watson Health.
16. Prevedelio LM, Erdal BS, Ryu JL, et al. Automated critical test findings identification and online notification system using artificial intelligence in imaging.
Radiology 2017;285:923-93.
17. Lehnis M. Can we trust AI if we don’t know how it works? BBC Online News, June 15, 2018. Accessed July 15, 2018.
18. Kahn CE. From images to actions: Opportunities for artificial intelligence in radiology.
Radiology 2017;285:719-720.
19. Kaplan J. Why we find self-driving cars so scary.
Wall Street Journal. May 31, 2018. Accessed May 31, 2018.
20. Ross C, Swetlitz I. Citing weak demand, IBM Watson Health to scale back hospital business. STAT+ June 15, 2018. Accessed June 15, 2018.
21. AMA Passes First Policy Recommendations on Augmented Intelligence. AMA June 14,2018. Accessed July 15, 2018.
22. Kirk IR, Sassoon D, Gunderman RB. The triumph of the machines.
J Am Coll Radiol 2018;15:587-588.
23. Schier R. Artificial intelligence and the practice of radiology: An alternative view.
J Am Coll Radiol 2018;in press. Accessed July 15, 2018.
24. Davenport TH, Dreyer KJ. AI will change radiology, but it won’t replace radiologists.
Harvard Business Review March 27, 2018. Accessed July 15, 2018
25. Yi PH, Hui FK, Ting DS. Artificial Intelligence in radiology: Collaboration is key.
J Am Coll Radiol 2018;15:781-783