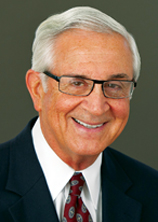
Summer 2016 - Vol. 11, No. 2
Can Science Be Trusted? Data Mining, P-Hacking, and Related Problems
Lawrence I. Bonchek, M.D., F.A.C.S., F.A.C.C.
Editor-in-Chief
I’m going to talk about statistics. That statement should be sufficient to send most readers to the next article or even to the next room, but please stay with me as this is an important subject.
DATA MINING — BY SCIENTISTS AND NEWSCASTERS
My last editorial
1 discussed Data Mining
* as one of the challenges of “Big Data.” Analysis of the massive amounts of data available in Electronic Medical Records or national data registries is bound to reveal many previously unsuspected associations among variables. Since association doesn’t mean causation, many of these newly discovered associations will be meaningless, and subsequent studies will be needed to sort the wheat from the chaff. Modern statistical methods and common sense will make it unnecessary to test every new association in a randomized clinical trial, but even if we avoid the most egregious absurdities, we will still wander down many blind alleys, while wasting time, energy, and resources.
A fundamental property of scientific knowledge is its self-correcting nature, but that process can only succeed if other investigators attempt to replicate the results of original studies. Unfortunately, career advancement depends upon original research, and that is where most scientists concentrate their energies. Replicative studies are poorly funded, and there are no awards for fact-checking. Further, as I noted last time, negative studies are less likely to see print, or — if they do — to achieve high visibility.
Another problem is the media’s penchant for their own special version of “mining” — monitoring the scientific literature for studies they can transform into striking headlines. Morning TV shows are a major source of news for many Americans, and their impact can be considerable, but scientific analysis is not their strong suit. Recently, morning show hosts proclaimed that chocolate in pregnancy was good for mother and child (by preventing pre-eclampsia). In fact, the report this claim was based on was only an abstract presented at a meeting,
2 and therefore not yet peer-reviewed. The study was not designed to assess whether chocolate had an incremental benefit in pregnancy, as it merely compared the uterine artery Doppler pulsatility index in two groups of pregnant women given chocolate with either high or low flavanols. Importantly, there was no control group that ate no chocolate.
Still, if this hyped report causes some pregnant women to eat a modest amount of chocolate unnecessarily, that won’t do anything more harmful than add a few calories to their diet. Further studies might clarify or even refute those media reports, but meanwhile the original reports will be online forever, with a title that implies benefit,
3 and the media will move on to distort the next sensational bit of “medical news,” which may have more serious implications.
P-HACKING AND RELATED ILLS
When data mining is used to uncover patterns in data that can be presented as statistically significant, without first devising a specific hypothesis as to the underlying causality, another harmful activity is also likely to rear its ugly head. So called p-hacking, or the process of sifting through combinations of variables by narrowing or expanding the data set analyzed until a desired result is achieved (usually p<.05) has arisen out of the nearly universal dependence on p<.05 as an indicator of statistical significance and a prerequisite for publication.
The website fivethirtyeight.com, which applies statistics to various aspects of daily life but most notably to sports and politics, vividly illustrates this phenomenon with an interactive graphic that analyzes whether the U.S. economy does better when Republicans or Democrats are “in power.”
3 In their illustration it is quite simple to prove that either Republicans or Democrats are better for the economy by tweaking the selection of variables that define “in power” (is it the party that controls the White House, Congress, the State Houses?) and “the economy” (is the best indicator the rate of inflation, unemployment, GDP, stock prices?). Making the selections involves exploiting what has been called “researcher degrees of freedom,”
4 or the choices researchers make as they conduct a study, such as which observations to record, which ones to compare, and which factors to control for.
Medical research is probably less prone to the problem of p-hacking than many other scientific disciplines, but in recent years it has embraced a statistical method that poses a specific challenge. Rather than mining data to unearth previously unknown associations, this method ironically requires us to have some idea in advance which variables are important enough to monitor. Because it is so difficult to accrue enough patients in randomized trials, there is a growing tendency to use large observational registries instead, and to apply propensity score matching (PSM) to match study groups of treated and untreated patients in important covariates. But whereas randomization makes no predictions or assumptions, and depends upon large numbers to provide comparable groups, PSM can only account for observed (and observable) covariates that are, in fact, being monitored. As I noted earlier, the choice of monitored variables can have an enormous influence on outcomes. We are thus increasingly dependent on knowing in advance (or thinking we know) which variables have some effect on outcomes, even if we don’t pretend to know what that effect is. Even without any malicious intent, factors that are unknown or cannot be observed are not accounted for in the matching procedure,
5 and any hidden bias due to these “latent variables” remains.
An interesting sidelight to these issues is the fact that medical peer reviewers and clinician readers probably have a less sophisticated understanding of statistical methods than do the readers of basic science journals. Moreover, the consequences of research errors in clinical studies have more immediate human impact.
Another problem that distinguishes medicine is that every clinician has his or her own clinical experience and biases. Since we process new evidence through the lens of what we already believe, confirmation bias can not only blind us to new information that contradicts our biases, but makes us too willing to believe faulty studies that confirm our beliefs.
In a related matter, as we go to press the New England Journal of Medicine has inaugurated a series of articles called The Changing Face of Clinical Trials, which will “examine the current challenges in the design, performance, and interpretation of clinical trials.” It appears from the editorial that announced the series in the issue of June 2, 2016 that its focus will be on the opportunities and challenges of integrating trials of comparative effectiveness into clinical care. We will follow the series with interest, and comment as appropriate.
IN THIS ISSUE
I only have space for two comments about the articles in this issue (see the inside front cover for summaries).
First, please note that in his section on Top Tips, Alan Peterson also draws attention to the hazard of relying on “p values.”
Second, in the article by Oyer and Breznak about drug shortages at LGH, it is worth noting that most of the listed drugs are generic injectables. Shortages of a commodity often involve pricing, as pointed out in the Upshot column by health economist Austin Frakt in the New York Times recently,
6 which brings a unique viewpoint to the challenge of drug shortages.
Though we usually complain about high drug prices, Frakt notes that for generic injectables, some drugs are not costly enough. Generic injectables are more difficult and costly to make than oral drugs, and they have low profit margins. They are prone to shortages because most generic injectables are produced by three or fewer companies. Any manufacturing problem at one of them is a major threat to the supply. If prices and profit margins would rise, other manufacturers would enter the market and prices would fall.
Since it can take years and cost hundreds of millions of dollars to get injectable production started and approved by regulators, Frakt notes that there is little incentive for new producers to enter the market. Apparently, there are fewer shortages in Europe, where generic injectable prices are higher.
* Data mining is a computational process that seeks patterns in large data sets by applying automatic or semi-automatic analytic methods that may come from artificial intelligence, machine learning, statistics, and database systems. The process hopes to extract previously unknown, interesting patterns which can then be further analyzed. In medicine, this process has unique appeal because it holds the possibility of arriving at useful clinical predictions that could directly impact patient management.
REFERENCES
1. Bonchek LI. Electronic medical records as a research tool: the opportunity and risk of big data. J Lanc Gen Hosp. 2016; 11:1-2.
2. Bujold E, Babar A, Lavoie E et al. High-flavanol chocolate to improve placental function and to decrease the risk of preeclampsia: a double blind randomized clinical trial. Abstract presented at the 2016 Annual Meeting of the Society for Maternal-Fetal Medicine, Atlanta Georgia.
3. http://fivethirtyeight.com/features/science-isnt-broken/
4. Simmons JP, Nelson LD, and Simonsohn U. False-Positive Psychology: undisclosed flexibility in data collection and analysis allows presenting anything as significant (May 23, 2011). Psychological Science, 2011. Available at SSRN:
http://ssrn.com/abstract=1850704
5. Garrido MM, et al. "Methods for constructing and assessing propensity scores". Health Services Research (Wiley) 2014; 49: 1701–20. doi:10.1111/1475-6773.12182. PMID 24779867.
6. http://www.nytimes.com/2016/05/31/upshot/drug-prices-too-high-sometimes-theyre-not-costly-enough.html